Transforming Data Management with AI Labeling Tools
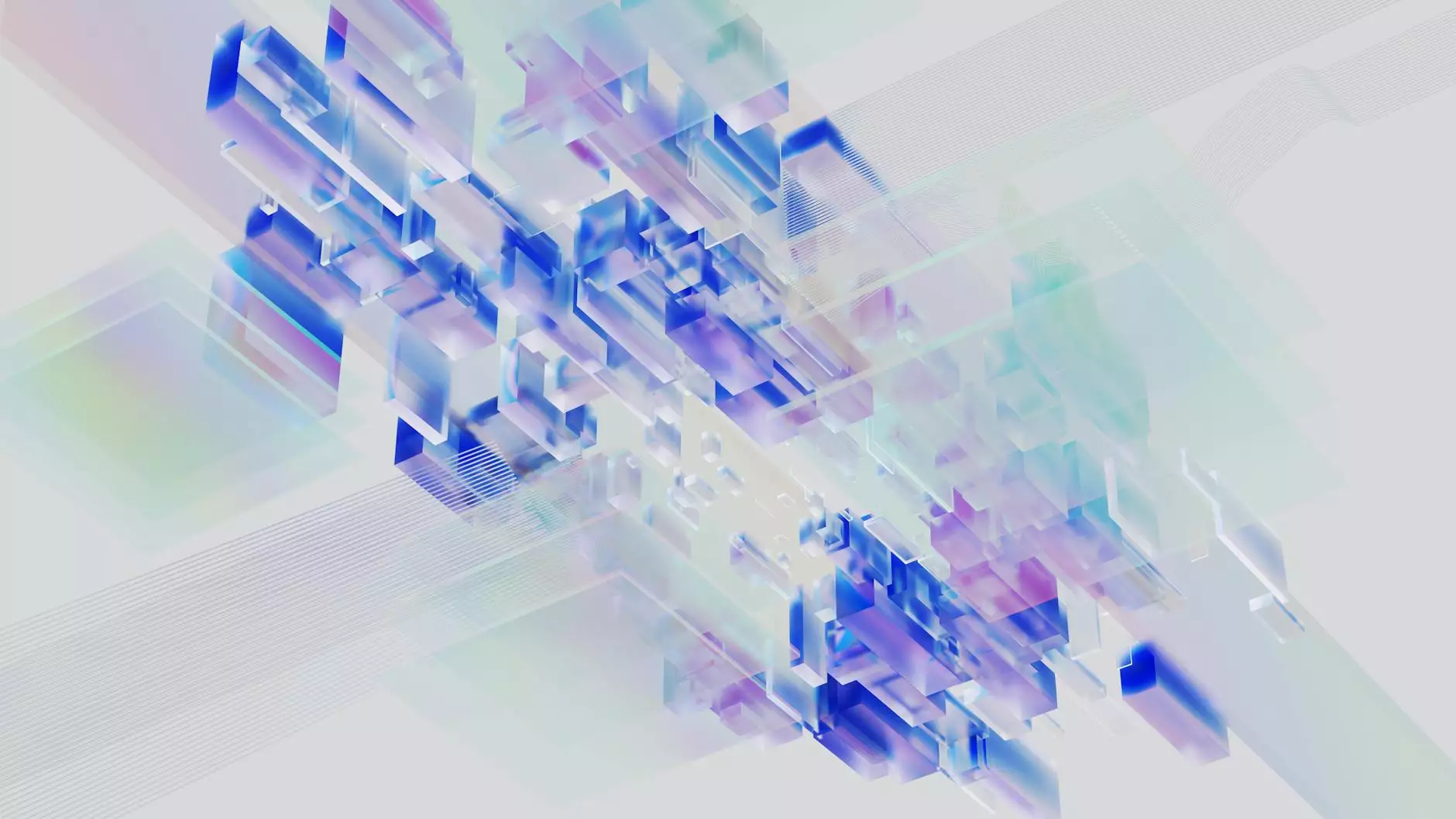
In the fast-evolving world of data management, the importance of AI labeling tools cannot be overstated. These powerful tools are revolutionizing how businesses approach data annotation, allowing them to streamline processes, reduce costs, and improve overall accuracy.
What Are AI Labeling Tools?
AI labeling tools serve as automated platforms designed to categorize and label data in a systematic manner. They leverage artificial intelligence algorithms to simplify the data annotation process, whether it’s for images, text, or videos. By automating repetitive tasks, these tools enable data scientists and businesses to focus on more strategic endeavors.
Why Businesses Need AI Labeling Tools
In a digital age where data is regarded as the new oil, the ability to efficiently process and label vast amounts of information can significantly influence a company's success. Here are several reasons why businesses should consider investing in AI labeling tools:
- Increased Efficiency: Traditional data labeling processes can be labor-intensive and time-consuming. AI tools can dramatically reduce the time taken to annotate data by automating mundane tasks.
- Cost-Effective Solutions: By decreasing the time spent on manual labeling, businesses can save significant costs associated with labor and resources.
- Improved Accuracy: AI-driven labeling minimizes human error, which can be pivotal in high-stakes industries, such as healthcare and finance.
- Scalability: As businesses grow, the amount of data they need to annotate scales with them. AI tools easily adapt to increased data volumes without compromising quality.
- Enhanced Collaboration: AI labeling tools often come with features that facilitate better teamwork and project management, ensuring that all stakeholders remain in sync.
Types of Data Supported by AI Labeling Tools
AI labeling tools excel in managing various types of data, including but not limited to:
1. Image Data Annotation
Image annotation involves tagging images with labels to help AI systems recognize patterns and objects. This can include:
- Bounding boxes: Drawing rectangles around objects in an image.
- Segmentation: Dividing an image into segments for a higher-level understanding.
- Classification: Assigning a category label to an entire image.
2. Text Data Annotation
Text annotation is pivotal for natural language processing applications. Common tasks include:
- Named Entity Recognition (NER): Identifying entities such as names, dates, and locations.
- Sentiment Analysis: Determining the sentiment expressed in a piece of text.
- Text Classification: Categorizing text into predefined labels.
3. Audio and Video Annotation
Multimedia data requires specialized annotation methods, which may include:
- Transcription: Converting spoken language into written text.
- Object Tracking: Identifying and following objects across video frames.
- Action Recognition: Labeling specific actions taking place in videos.
How AI Labeling Tools Work
Understanding how AI labeling tools function can help businesses maximize their use. Here’s an overview:
1. Data Ingestion
The first step in the labeling process is data ingestion, where raw data is uploaded into the AI labeling tool. Most modern tools support various formats, such as images, text files, and video formats, ensuring flexibility in data management.
2. Pre-processing
Once the data is ingested, pre-processing occurs. This involves cleansing the data, removing irrelevant information, and formatting data for efficient tagging. Pre-processing ensures that the data is ready for annotation.
3. Automated Labeling
During the automated labeling phase, the AI algorithms analyze the data based on predefined rules or machine learning models. This process ensures that the data is labeled accurately and consistently without excessive human intervention.
4. Human Validation
Despite automation, human validation remains a crucial step. Human annotators review the AI-generated labels to ensure quality and correctness. This collaboration between AI and humans enhances the overall accuracy of the labeled data.
5. Output Generation
Finally, the validated data is outputted in a format suitable for training AI models. Common formats include CSV files, JSON, and XML, depending on the requirements of the machine learning applications.
Key Benefits of Utilizing AI Labeling Tools
The implementation of AI labeling tools provides businesses with numerous advantages, including:
1. Improved Data Quality
AI tools ensure consistency throughout the labeling process, thereby enhancing the quality of the data collected. High-quality data leads to better performing AI models.
2. Accelerated Project Timelines
With the ability to process and label data at unprecedented speeds, companies can achieve faster turnaround times on projects, allowing them to stay competitive in the marketplace.
3. Competitive Advantage
Organizations that leverage advanced AI labeling tools gain a significant edge over their competitors by enabling quicker insights and faster innovations.
4. User-Friendly Interfaces
Many modern AI labeling tools come equipped with user-friendly interfaces that simplify the data annotation process. This accessibility allows teams without extensive technical backgrounds to effectively employ these tools.
Choosing the Right AI Labeling Tool for Your Business
With numerous AI labeling tools available in the market, choosing the one that aligns with your business needs is crucial. Here are factors to consider:
- Customization: Ensure the tool allows for customization to suit your specific data types and labeling requirements.
- Integration Capabilities: The tool should integrate seamlessly with your existing data management systems and workflows.
- Scalability: As your data needs grow, the tool should be able to scale accordingly without loss of performance.
- Support and Training: Look for vendors that offer comprehensive support and training resources to assist your team in utilizing the tool effectively.
Future Trends in AI Labeling Tools
The landscape of AI labeling tools is continuously evolving. Future trends may include:
1. Greater AI Integration
As artificial intelligence continues to advance, we can expect even smarter labeling tools that learn and adapt over time, increasing their predictive accuracy and efficiency.
2. Enhanced User Interfaces
Improvements in user interface design will make AI labeling tools more intuitive, enabling non-technical users to participate in the labeling process effectively.
3. Ethical Considerations
As data privacy and ethical concerns grow, future labeling tools will likely incorporate features that ensure compliance with data protection regulations while maintaining transparency in the labeling process.
4. Collaborative Labeling Solutions
New platforms may emerge that facilitate collaboration among teams globally, enhancing the ability to annotate data in real-time, thus improving project efficiency.
Conclusion
The rise of AI labeling tools signifies a transformative shift in how businesses manage and utilize data. By harnessing these advanced technologies, companies can streamline their annotation processes, reduce costs, and enhance the quality of their outputs. As we look to the future, it’s clear that the role of AI labeling tools will only continue to grow—making them an essential investment for businesses aiming to thrive in a data-driven world.
Discover KeyLabs.ai
At KeyLabs.ai, we specialize in providing cutting-edge data annotation tools and data annotation platforms that can transform your data labeling processes. Explore our range of solutions and see how we can help you take your data management efforts to new heights.